AI’s impact: Canadian and US researchers are proposing a four-pillar structure for the social and environmental effects of AI. Researchers from the Montreal AI Ethics Institute, McGill University, Carnegie Mellon, and Microsoft have co-published a paper proposing a four-pillar framework that combines socio-technical measures under an approach called ‘SECure’ in an attempt to build more responsible artificial intelligence (AI) technologies. Such initiatives take AI’s eco-social obligation and effect into account.
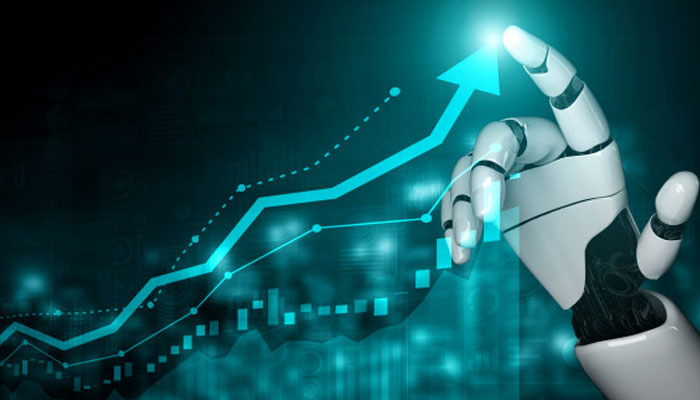
“In a world increasingly dominated by AI applications, an understudied aspect is the carbon and social footprint of these power-hungry algorithms that require copious computation and a trove of data for training and prediction. While profitable in the short-term, these practices are unsustainable and socially extractive from both a data-use and energy-use perspective,” write the authors while introducing the paper. The SECure methodology aims to tackle understated concerns of sustainability, privacy and accountability across four pillars: compute-efficient machine learning, federated learning, data sovereignty, and a LEEDesque certificate.
Recently, Massachusetts University research has shed light on how AI’s impact AI models training leads to large carbon footprints. The research revealed how training an AI model has about 626,000 pounds of carbon dioxide emissions — more than five times the emission a typical US car would lead to in a lifetime! “While profitable in the short-term, these practices are unsustainable and socially extractive from both a data-use and energy-use perspective,” states the paper’s abstract.
SECure’s first pillar is computationally effective machine learning. Training an AI model is usually very costly, requiring access to sophisticated technologies, databases, and is observed for students and researchers associated with affluent universities and organizations to pursue.
This creates a social exclusivity that excludes the inspired people from the field. Therefore, the researchers argue that “If AI is more compute-efficient to the point where it requires only a laptop or other relatively obtainable hardware, the field of AI may become much more accessible. Compute-efficient machine learning could thereby have a sizable social impact.”
The second pillar of SECure implies the use of federated learning methods as a method to do on-device training and inference of ML models. “The purpose of utilizing this technique is to mitigate risks and harm that arises from the centralization of 8 data, including data breaches and privacy intrusions,” argue the researchers. A secondary advantage, as researchers have pointed out, also included potentially decreasing carbon impacts, if renewable sources produce the electricity needed to perform computations. We do, however, answer the questions about ‘economies of scale’.
Data sovereignty is SECure’s third pillar, relating to the principle of strong data ownership and granting individuals autonomy over how, with their permission and how they see appropriate, their data is used. “In the domain of machine learning, especially where large data sets are pooled from numerous users, the withdrawal of consent presents a major challenge,” the researchers wrote.
“Specifically, there are no clear mechanisms today that allows for the removal of data traces or of the impacts of data related to a user.. without requiring retraining of the system.” For example, indigenous people view their data differently and ask that it be maintained on indigenous land, used and processed in ways that are consistent with their values.
Read More: artificial intelligence helping in online education
SECure’s final pillar is LEED-esque certification. The certificate is influenced by Energy and Environmental Design Leadership. The researchers are suggesting certification which would include a standard metric. This will allow various AI users to determine the level of functionality of the AI system, as opposed to other AI systems.
AI’s impact The researchers are hopeful that with SECure being implemented on a scale, various sectors of the community-academics, students, customers, and investors will have the power to demand greater transparency on socio-environmental impacts.
“Responsible AI investment, akin to impact investing, will be easier with a mechanism that allows for standardized comparisons across various solutions, which SECure is perfectly geared toward,” the coauthors wrote. “From a broad perspective, this project lends itself well to future recommendations in terms of public policy.”